LSA will take place virtually over the course of two days. Oral sessions will be hosted on Zoom, and can be joined
via the link below.
Zoom Link: https://us06web.zoom.us/j/88330584074?pwd=R2poa1A1M3VkcDhWZ2JQOCtCKzdWdz09
In addition, LSA is hosting two joint poster sessions with the ALA and GAIW workshops, which are also taking place
at AAMAS 2022. Each poster session will take place in the Gather Town space shared below:
Gather Town Link: https://app.gather.town/events/O8p6uZQ3G1EJELYsXH2v
Please find detailed information regarding the workshop schedule below. Please note that all times and dates are listed
in Auckland time (UTC+12).
Tuesday, May 10
Time (GMT+12) | Subject | Authors | Title |
---|---|---|---|
02:00-02:55 | Poster Session 1 | ||
03:00-03:20 | Paper Spotlight | Xiaotie Deng, Xinyan Hu, Tao Lin and Weiqiang Zheng | Nash Convergence of Mean-Based Learning Algorithms in First Price Auctions |
03:20-03:40 | Paper Spotlight | Vineet Nair, Ganesh Ghalme, Inbal Talgam-Cohen and Nir Rosenfeld | Strategic Representation |
03:40-04:00 | Paper Spotlight | Tal Lancewicki, Aviv Rosenberg and Yishay Mansour | Cooperative Online Learning in Stochastic and Adversarial MDPs |
04:00-05:00 | Invited Talk | Panayotis Mertikopoulos | Limits and Limit Points of Game-Theoretic Learning |
05:00-05:20 | Paper Spotlight | Gabriel Andrade, Rafael Frongillo and Georgios Piliouras | No-Regret Learning in Games is Turing Complete |
05:20-05:40 | Paper Spotlight | Martino Bernasconi, Federico Cacciamani, Simone Fioravanti, Alberto Marchesi, Nicola Gatti and Francesco Trovò | Exploiting Opponents Subject to Utility Constraints in Extensive-Form Games |
06:15-07:15 | Poster Session 2 |
Wednesday, May 11
Time (GMT+12) | Subject | Authors | Title |
---|---|---|---|
03:00-04:00 | Invited Talk | Amy Greenwald | No-Regret Learning in Extensive-Form Games |
04:00-04:20 | Paper Spotlight | Andrew Estornell, Sanmay Das, Yang Liu and Yevgeniy Vorobeychik | Unfairness Despite Awareness: Group-Fair Classification with Strategic Agents |
04:20-04:40 | Paper Spotlight | Keegan Harris, Valerie Chen, Joon Sik Kim, Ameet Talwalkar, Hoda Heidari and Z. Steven Wu | Bayesian Persuasion for Algorithmic Recourse |
04:40-05:00 | Paper Spotlight | Tosca Lechner and Ruth Urner | Learning Losses for Strategic Classification |
05:00-05:20 | Paper Spotlight | Lirong Xia | How Likely A Coalition of Voters Can Influence A Large Election? |
05:20-05:40 | Paper Spotlight | Fan Yao, Chuanhao Li, Denis Nekipelov, Hongning Wang and Haifeng Xu | Learning from a Learning User for Optimal Recommendations |
05:40-06:00 | Paper Spotlight | Keegan Harris, Daniel Ngo, Logan Stapleton, Hoda Heidari and Z. Steven Wu | Strategic Instrumental Variable Regression: Recovering Causal Relationships From Strategic Responses |
Invited Talks
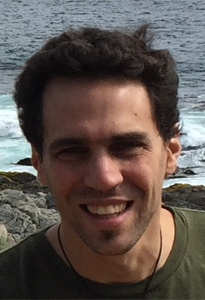
Panayotis Mertikopoulos
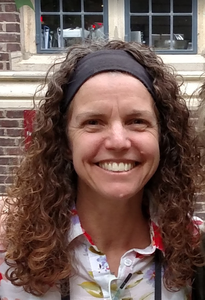
Amy Greenwald
Speaker: Panayotis Mertikopoulos, French National Center for Scientific Research (CNRS)
Title: Limits and Limit Points of Game-Theoretic Learning [Video]
Talk Abstract
Does learning from empirical observations in a game-theoretic setting converge to a Nash equilibrium? And, if so, at what rate? A well-known impossibility result in the field precludes the possibility of a "universally positive" answer - i.e., the existence a dynamical process which, based on player-specific information alone, converges to Nash equilibrium in all games. In view of this, we will instead examine the equilibrium convergence properties of a class of widely used no-regret learning processes which includes the exponential weights algorithm and the “follow the regularized leader” family of methods, their optimistic variants, extra-gradient schemes, and many others. In this general context, we establish a comprehensive equivalence between the stability of a Nash equilibrium and its support: a Nash equilibrium is locally stable and attracting if and only if it is strict (i.e., each equilibrium strategy has a unique best response). We will also discuss the robustness of this equivalence to different feedback models - from full information to bandit, payoff-based feedback - as well as the methods' rate of convergence in terms of the underlying regularizer and the type of feedback available to the players.
Speaker Bio
Panayotis Mertikopoulos is a principal researcher at the French National Center for Scientific Research (CNRS). Before joining CNRS, he received his undergraduate degree in physics from the University of Athens, his MSc and MPhil in mathematics from Brown University, and his PhD also from the University of Athens. He has since spent a year at École Polytechnique in France as a postdoctoral researcher, and has held visiting positions as an invited professor at the University of Rome, UC Berkeley, and EPFL. His research interests span the interface of game theory, learning and optimization, with a special view towards their applications to machine learning, operations research, and network theory. He is especially interested in the equilibrium convergence properties of multi-agent learning algorithms and dynamics, their rate of convergence (when they converge), and the type of off-equilibrium behavior that may arise (when they do not).
Speaker: Amy Greenwald, Brown University
Title: No-Regret Learning in Extensive-Form Games [Video]
Talk Abstract
The convergence of \Phi-regret-minimization algorithms in self-play to \Phi-correlated-equilibria is well understood in normal-form games (NFGs), where \Phi is the set of deviation strategies. This talk investigates the analogous relationship in extensive-form games (EFGs). While the primary choices for \Phi in NFGs are internal and external regret, the space of possible deviations in EFGs is much richer. We restrict attention to a class of deviations known as behavioral deviations, inspired by von Stengel and Forges' deviation player, which they introduced when defining behavioral correlated equilibria (BCE). We then propose extensive-form regret minimization (EFR), a regret-minimizing learning algorithm whose complexity scales with the complexity of \Phi, and which converges in self-play to BCE when \Phi is the set of behavioral deviations. Von Stengel and Forges, Zinkevich et al., and Celli et al. all weaken the deviation player in various ways, and then derive corresponding efficient equilibrium-finding algorithms. These weakenings (and others) can be seamlessly encoded into EFR at runtime, by simply defining an appropriate \Phi. The result is a class of efficient \Phi-equilibrium finding algorithms for EFGs.
Speaker Bio
Amy Greenwald is Professor of Computer Science at Brown University in Providence, Rhode Island. Her research focuses on game-theoretic and economic interactions among computational agents, applied to areas like autonomous bidding in wireless spectrum auctions and ad exchanges. Before joining Brown, Greenwald was a postdoctoral researcher at IBM's T.J. Watson Research Center, where her "Shopbots and Pricebots" paper was named Best Paper at IBM Research. Her honors include the Presidential Early Career Award for Scientists and Engineers (PECASE), a Fulbright nomination, and a Sloan Fellowship. Finally, Greenwald is active in promoting diversity in Computer Science, leading multiple K-12 initiatives in which Brown undergraduates teach computer science to Providence public school students.